In the complex landscape of financial services, particularly in lending, understanding borrower behavior is critical. Traditional data sources like credit bureaus provide a baseline for evaluating credit risks, including delinquency rates. However, alternative data sources, such as transaction data from Envestnet® | Yodlee®, offer a novel perspective that can augment and refine these assessments, especially in contexts where direct data on delinquencies are sparse or unavailable.
The Framework for Inferring Delinquency
Using Yodlee’s extensive deidentified transaction data, we developed a framework to infer loan delinquency rates from transaction patterns. This approach primarily focuses on the repayment behavior. Here's how we break it down:
- Repayment intervals: We compute the mean interval between successive payments for each borrower, which helps in identifying payment regularity and potential signs of distress.
- Classification of payment timeliness: Payments are categorized into three statuses: on-time, early, and late. This classification helps in detecting deviations from the expected payment schedules, which could indicate emerging financial distress.
- Cohort analysis: The analysis applies various controls and filters to focus on specific borrower profiles, such as new borrowers, borrowers with multiple loans, and those meeting minimum transaction activity thresholds.
Data and Controls
To ensure the reliability and applicability of our findings, the following filters and controls were implemented:
- Net-new borrowers: Filtering out long-standing borrowers to focus on newer loan accounts.
- Multiple loans: Excluding borrowers juggling multiple loan responsibilities for clearer insights.
- Activity thresholds: Only including borrowers with consistent transaction activities.
- Exclusion of lump sum payments: These are not considered to avoid skewed interval data.
Empirical Validation
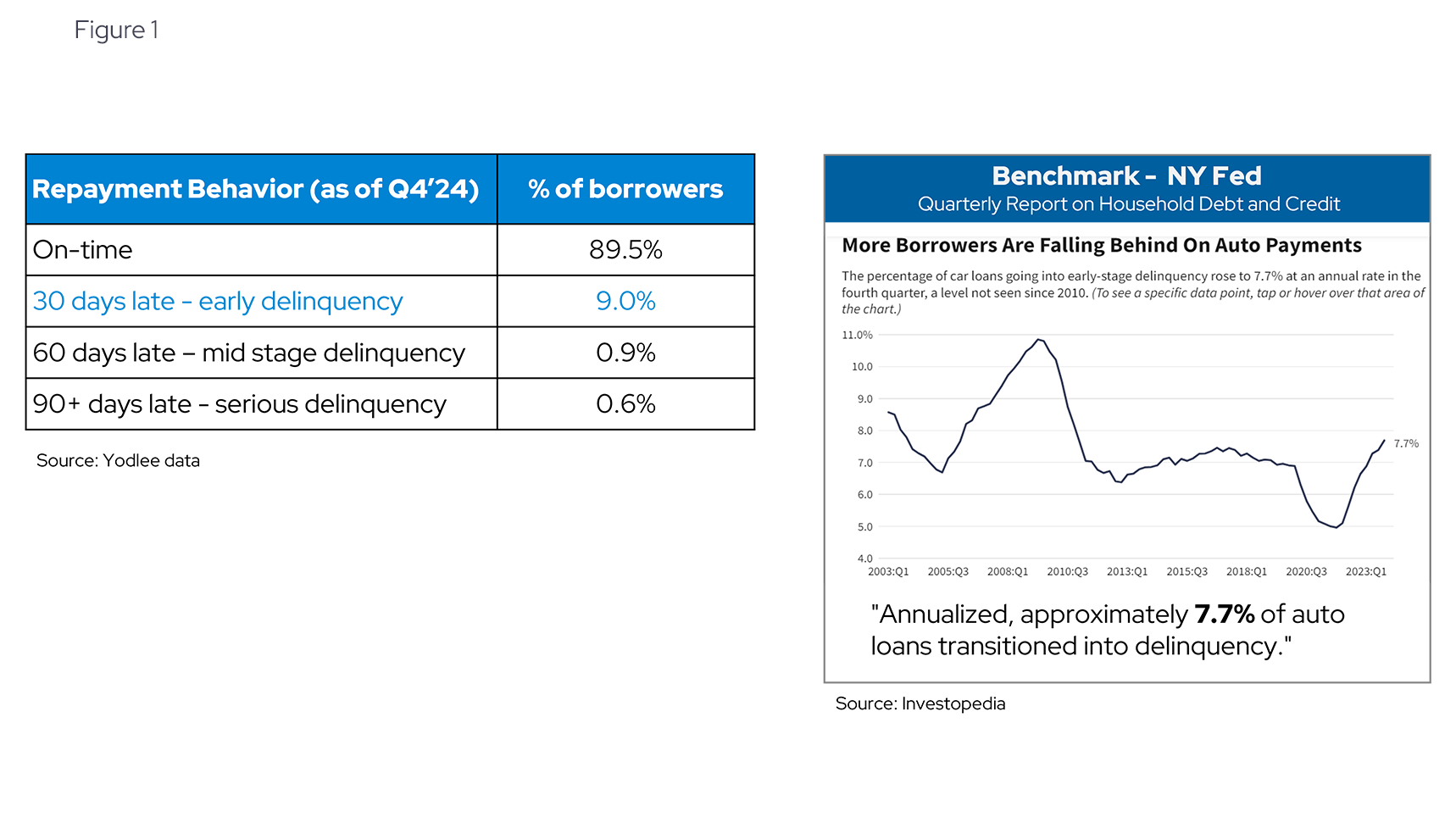
We analyzed the transaction data of 800,000 individuals with active auto loans from Yodlee’s data, which includes several financial institutions. To adjust for potential discrepancies due to bank posting delays or holidays, additional buffer days were considered.
Comparing our findings with the New York Federal Reserve’s report, which uses a more traditional and representative set of data, we observed a remarkable closeness in the results: the Federal Reserve Bank of New York reports a 30-day delinquency rate of 7.7%, whereas our analysis shows a rate of 9.0% (see Figure 1). This proximity validates our approach, considering the different nature of the data sources involved.
Applicability Across Products and Lenders
The methodology developed from Yodlee’s transaction data is not only limited to auto loans but is also applicable to other products like mortgages and credit cards. This versatility allows lenders across different segments to utilize this analysis to gauge the health of their portfolios.
Using Transaction Data to Inform Credit Decisions
The use of Yodlee’s transaction data to infer auto loan delinquency rates represents a significant advancement in credit analysis. It offers lenders an additional tool to monitor and manage credit risk proactively, using up-to-date transaction data to capture nuances that traditional data sources might overlook. As financial markets continue to evolve, integrating such innovative approaches will be crucial for maintaining a competitive edge and enhancing the accuracy of credit risk assessments. This not only benefits lenders but also contributes to a more stable and responsive financial ecosystem.
Want to leverage alternative lending data to create competitive offers, help minimize risk, and serve a wider range of consumers?
Learn more about Envestnet | Yodlee Credit Accelerator
About Envestnet | Yodlee Merchant, Retail, Shopper, and Spend Insights
Envestnet | Yodlee Merchant, Retail, Shopper, and Spend Insights utilize de-identified transaction data from a diverse and dynamic set of data from millions of accounts to identify patterns and context to inform spending and income trends. The trends reflect analysis and insights from the Envestnet | Yodlee data analysis team. By combining data with intelligence – connecting vast amounts of actual de-identified shopping data with state-of-the-art analytics and machine learning – Envestnet | Yodlee provides visibility into a large set of shopping daily purchase behavior including, but not limited to, transactions, customer lifetime values, and merchant/retailer shares.
To learn more about Envestnet | Yodlee Merchant and Retail Insights and get a free demo, please contact an Envestnet | Yodlee sales representative.