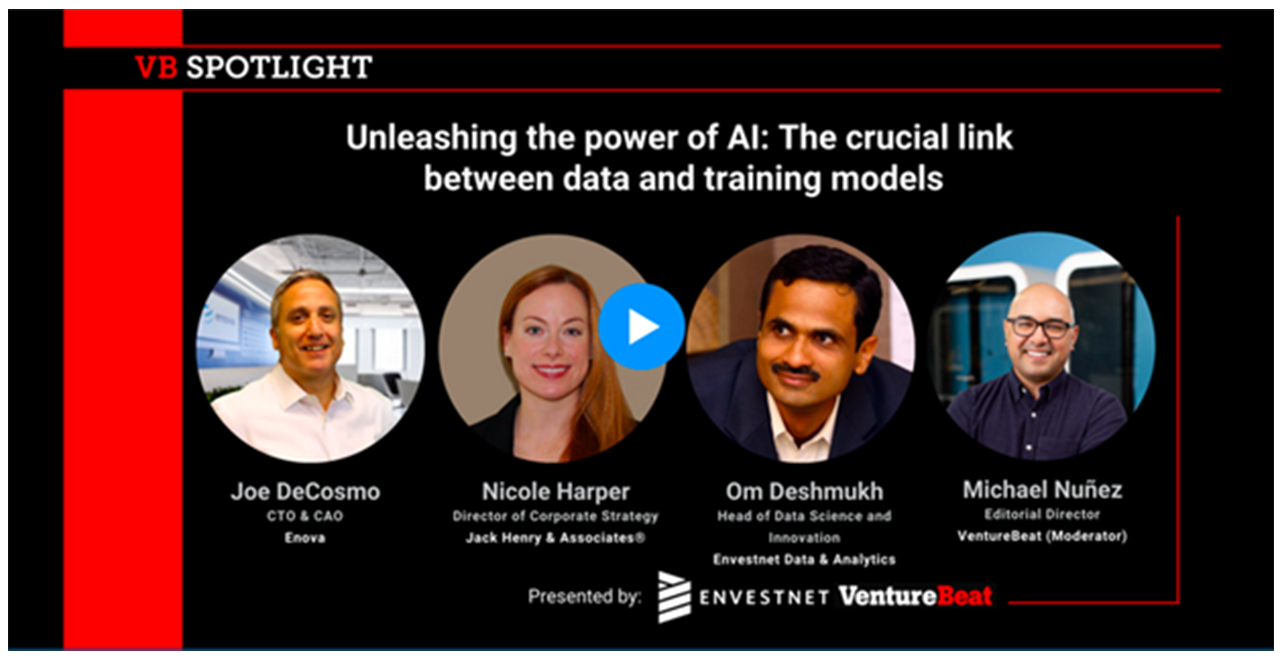
Data-driven strategies like AI, machine learning, and analytics are transforming financial services and driving hyper-personalization at scale – which is taking product development, marketing, and customer satisfaction to new heights.
From helping customers budget for irregular expenses to identifying clients who are ready for home loans or credit card upsell opportunities, there are unlimited use cases that AI and ML can power for financial service providers. Om Deshmukh, head of data science and innovation at Envestnet | Yodlee, outlined some of these use cases in an interview with VentureBeat.
Deshmukh highlighted how at Envestnet | Yodlee, we’ve used data-driven machine learning to help financial service providers identify which customers are likely to be negatively impacted by inflation and determine which set of users would benefit from a warning and which customers should be offered loan repayment flexibility.
We’ve also used data analytics to help a client target customers needing assistance finding their footing after the pandemic. Our client was able to identify those who were more likely to take a delayed vacation, who’d find an offer for a credit card with airline points attractive. They also uncovered other patterns and behaviors, such as with customers who were interested in going out to eat vs ordering takeout.
“What’s truly transformative is how these technologies have unlocked even deeper layers of hyper-personalization,” says Deshmukh. “Financial services companies have always had a bonanza of data at their fingertips, but now it’s possible to process it at scale, turning it into structured, tagged, and enriched data that can accelerate innovative product development and enable targeting all the way down to the individual customer.”
Data-driven insights can also enable a financial institution to compare itself with its own peer groups, or local or national macroeconomic situations, and set benchmarks. Within a few weeks of the major bank fallout, we helped FI clients benchmark themselves against other FIs to assess their cash inflow and outflow status in real-time and course correct as needed.
3 factors driving AI and ML innovation
Deshmukh explained how the turning point for this new level of data-driven innovation came about due to the convergence of three factors. First, the amount of data that is available has increased multifold. Approximately 328.77 million terabytes of data are generated each day, and 181 zettabytes of data are expected to be generated in the year of 2025, according to some estimates.
Second, access to pre-trained models has been democratized. A few years ago, all of these machine-learning models were proprietary. They weren’t exposed, and they weren’t easily accessible to organizations without the time, money, and expertise to invest in developing them. But today, they’re available to any organization.
And the third factor, which Deshmukh emphasized is often overlooked but is every bit as important, is access to the computing power necessary to run these models. Cloud has made computing far more affordable and attainable so that companies can run their models without the worry of computational and cost barriers.
Data breadth and quality are critical for optimal results
AI data models are only as good as the data used to feed and optimize them, however. Deshmukh and other thought leaders expanded on this point in the VB Spotlight webinar: Unleashing the Power of AI: The Crucial Link Between Data and Training Models. You can watch it on demand here.
In the webinar, panelists discussed how data enrichment, which eliminates the garbage-in garbage-out problem, is essential since insights are only as valuable or as accurate as the data you’re working with. Along with making data more accurate, data enrichment adds critical context to transactions, which can drive specific insights.
AI and machine learning algorithms also need access to a broad array of data sources. Using a limited number of sources that aren’t representative of your user base or use case can result in substantial bias in the system.
Success depends on nailing down your objective before using AI, whether you aim to improve the customer experience, increase efficiency, or drive revenue.
Starting with the full financial picture
By aggregating and enriching transactions, a detailed customer portrait can be created. Every step in a consumer’s daily financial journey adds another piece of important information.
For example, a customer may use one debit card at Walmart, another at Starbucks, and a third at their ATM. Each of these transactions can be pulled together to display a holistic picture that enables financial service providers to realize new personalization opportunities, deliver data-driven lending strategies, develop truly precise targeting, and more.
To explore more use cases for AI and analytics, discover how data can make or break an algorithm, and learn how to increase the power of your AI solution, watch Unleashing the Power of AI.